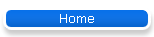
| |
Effing the ineffable:
An engineering approach to consciousness
Steve Grand
Abstract
This article supports the idea that synthesis, rather than analysis, is the
most powerful and promising route towards understanding the essence of brain
function and consciousness – at least, to the extent that consciousness is
capable of being understood at all. It discusses ‘understanding by doing’,
outlines a methodology for the use of deep computer simulation and robotics in
pursuing such a synthesis, and then briefly introduces the author’s ongoing,
long-term attempt to build a neurologically plausible and hopefully at least
sub-conscious being, who he hopes will eventually answer to the name of
Lucy.The Easy Problem of ‘as’
According to the Shorter Oxford English Dictionary the word ‘ineffable’ has a
number of meanings, one of which seems inexplicably to have something to do with
trousers. More usually, the term is used to describe a name that must not be
uttered, as in the true name of God, but ineffable can also mean something that
is incapable of being described in words. Consciousness, in the sense of
what it really means to be a subjective ‘me’, rather than an objective ‘it’,
might turn out to be just such a phenomenon. It may be that language and indeed
mathematics are fundamentally insufficient or inappropriate as an explanatory
tool for describing consciousness, and David Chalmers’ Hard Problem [Chalmers
1995] might in the end prove to be quite ineffable.
Nevertheless, there are other means to achieve understanding, besides abstract
symbolic description. After all, even apparently trivial concepts are often
inaccessible to verbal explanation. I find myself completely unable to define
the simple word ‘as’, for example, and yet I am able to use it accurately in
novel contexts and can interpret its use by others, so therefore can be said to
understand it. My understanding of ‘as’ is demonstrated operationally.
In linguistic terms, an operational understanding involves
the ability to use or interpret a word or expression successfully in a wide
variety of contexts. For an engineer, on the other hand, an operational
understanding is demonstrated by the ability to build something from first
principles and make it work. If I can build an internal combustion engine
without slavishly copying an existing example, then I can legitimately be said
to understand how one works, even if I am quite incapable of expressing that
understanding in words or equations.
An operational definition of consciousness might exist if
we were able to build an artificial brain (attached to a body, so that it could
perceive, act and learn), which reasonably sceptical people were willing to
regard as conscious (that is, they should be just as willing to ascribe
consciousness to the machine as they are to ascribe it to other human beings).
Of course, simply emulating consciousness, by building a highly
sophisticated puppet designed to fool the observer, should not be sufficient,
and here we see a danger inherent in the famous Turing Test for intelligence
[Turing 1950], since it turns out to be quite easy to fool most of the people
for at least some of the time.
Failing the Turing Test isn’t hard, but nothing should ever
really be regarded as having passed it, since it would need to put on a
convincing performance for an indefinite period if we are to differentiate
between true general intelligence and a finite set of pre-programmed responses.
So for an artificial brain to be able to show that we have obtained an
operational understanding of consciousness, it should also be built according to
a rational set of principles, which are accessible to observers, and
should implicitly demonstrate how those principles give rise to the behaviour
that is regarded as conscious. In other words, it must encapsulate a theory at
some level, even if the totality of that theory can’t be abstracted from its
implementation and described in words or simple equations. In particular, the
conscious-seeming behaviour must demonstrably emerge from the mechanism
of the machine, rather than be explicitly implemented in it, just as a model of
an internal combustion engine would be expected to drive itself, not be turned
by a hidden electric motor.
I shall return to the distinction between emulation and
replication in a moment. For now, I simply want to assert that synthesis is a
genuine, powerful method for achieving understanding, and a respectable
alternative to analysis, despite its low status in the reductionistic toolkit of
science. Strictly, synthesis of an apparently conscious machine would only
constitute an answer to Chalmers’ Easy Problems, of course – a description of
the mechanism by which consciousness arises, rather than an explanation of the
nature of subjectivity. Nevertheless, it may be as close as one can get, and it
may still demonstrate a higher understanding in an operational sense, in a way
that a written theory can’t do.
At the very least, attempts to synthesise consciousness
artificially might offer an answer to a Fairly Hard Problem, which lies
somewhere between those set by Chalmers. Theories of consciousness (or at least
heartfelt beliefs about it) tend to lie at one extreme or the other of the
organisational scale. To some, myself included, consciousness is clearly a
product of high levels of organisation: it is an emergent phenomenon that
perhaps did not exist anywhere in the universe until relatively recently and
could not exist at all until the necessary precursors had arisen. To me, a
conscious mind is a perfectly real entity in its own right and not some kind of
illusion, but it is nevertheless an invention of a creative universe. Yet for
many, consciousness belongs at the very opposite end of the scale, as something
fundamental – something inherent in the very fabric of the universe. Overt
Cartesian dualism lies at this extreme, as does the science fiction notion of a
‘life force’ and Roger Penrose’s rather more sophisticated retreat into the
magic of quantum mechanics [Penrose 1989]. Consciousness is clearly not a
property of medium levels of organisation, but at which of these two extremes
does it really lie?
To my mind the ‘fundamentalists’ don’t have a leg to stand
on, for simple philosophical reasons. One only has to ask them whether they
think their elemental Cartesian goo is simple or complex in structure. If it is
complex, then it is surely a machine, since consciousness is a property of its
organisation, not its substance. But why add the complication of a whole
new class of non-physical machines when we haven’t yet determined the limits of
physical ones? If, on the other hand, they think their fundamental force or
elemental field is unstructured and shapeless, as Descartes clearly envisaged
it, then how do they explain the fact that it has complex properties? How can a
shapeless, sizeless, timeless ectoplasm vary from place to place and moment to
moment? Why does it give rise to intricate behaviour? A force can push, but it
can’t choose to push one minute and pull the next, and then do something quite
different at other times. Moreover, if consciousness resides in some universal
goo, then why did it have to wait around until the evolution of complex brains
before its effects became apparent? Why does it require such specialised (and
delicate) equipment?
Despite the absurdity of it, many people cling on to
essentially Cartesian views, even if they don’t admit to it, but success at
creating artificial consciousness would certainly tip the balance in favour of
the emergent viewpoint, since no fairy dust would have been added to achieve the
result. Failure, of course, would prove nothing.
Returning to the more practical but still extremely
important and challenging ‘Easy Problem’ of the mechanism that underlies
conscious thought, I contend that synthesis might not simply be a useful
alternative to analysis, but may prove to be the only way in which we
might come to understand the brain. The brain may be genuinely irreducible. It
might be incapable of abstraction into a simple model without losing something
essential, and its basic principles of operation might not be deducible from its
parts. Neuroscience tries to understand the brain by taking it to pieces, and
although this is a vital part of any attempt to understand things, such
reductionism may not be sufficient in itself to give us the answer. At its
worst, this is like trying to understand how a car works by melting one down and
examining the resulting pool of molten metal. It isn’t steel that makes a car
go; it is the arrangement of steel, and the principles that underlie this
arrangement. Taking complex non-linear systems to pieces to see how they work
often leaves one with a large pile of trees but no sign of the wood.
It may be that the brain has no basic operating principles
at all, of course. To many observers the brain, even the notably rather regular
structure of the cerebral cortex, is an amazingly complicated hotchpotch. They
see the brain as an ad hoc structure patched together by evolution, in
which each functional unit has its own unique way of doing things. Explicitly or
implicitly, many people also regard the brain as a hard-wired structure (despite
overwhelming evidence to the contrary), and their focus is therefore on the
wiring, rather than the self-organising processes that give rise to the wiring.
In truth, the latter may be relatively simple even though the former is
undoubtedly complex, more on which below.
This attitude to the undeniable complexity of the brain
reminds me strongly of the way people interpreted the physical universe, before
Isaac Newton. The way that planets move in their orbits seemed to have nothing
in common with the way heat flows through a material or a bird stays in the air
– each seemed to require its own unique explanation. And then along came Newton
with three simple observations: if you push something it will move until
something stops it; the harder you push it, the faster it will go; and when you
kick something your toe hurts because the thing kicks you back just as hard.
Newton’s three laws of motion brought dramatic coherence to the world. Suddenly
(or at least as suddenly as the implications began to dawn on people), many
aspects of the physical universe became amenable to the same basic explanation.
I suggest that one day someone will uncover similar basic operating principles
for the brain, and as the relevance of these principles emerges, so the brain’s
manifold and apparently disparate, inexplicable properties will all start to
make sense.
I should emphasise that the above statement is not
intended as a defence of ultra-reductionism. The existence of elegant,
fundamental principles of operation does not ‘explain away’ the brain or
consciousness – the principles are absolutely no substitute for their
implementation, as I hope to amplify in the following section.
I submit that trying to build a brain from scratch is more
likely to uncover these basic operating principles than taking brains to pieces,
or studying vision in isolation from hearing, or cortex in isolation from
thalamus. As an engineer, one is faced directly with the fundamental questions –
the basic architecture comes first, and the details follow on from this. To
start with the fine details and try to work backwards towards their common
ground can quickly become futile, as the facts proliferate and the inevitable
need for specialisation causes people’s descriptive grammars to diverge rather
than converge.
Deep simulation
One of the best reasons to use an engineering approach, and especially computer
simulation, as a means to understand the brain is that computers can’t easily be
bluffed. Philosophers can wave their hands, and psychologists can devise
abstract theories that appear to fit the facts, but such explanations require
interpretation by human beings, and people are extremely good at lying to
themselves or skipping lightly over apparently small details that would crush a
theory if they were given sufficient attention. Take the instructions on a
packet of shampoo: ‘wet hair, massage shampoo into scalp, rinse off and repeat’.
The author of these instructions was presumably quite satisfied as to their
accuracy and sufficiency, and millions of people have since read them and
believed that they were acting exactly as instructed. Yet give those
instructions to a computer and it would never get out of the shower, since they
contain an infinite loop. Human beings know that they should only repeat the
instructions once, and so don’t even notice that the ‘theory’ embedded in the
algorithm is incomplete. Computers, on the other hand, do exactly what they are
told, even if this is not what you thought you were telling them.
But if computer synthesis is ever to succeed in demonstrating that consciousness
is an emergent phenomenon with a mechanistic origin, or even if it is only to
shed light on the operation of natural brains, certain mistakes and
misapprehensions need to be addressed regarding its use. I’ve met at least one
prominent neuroscientist who flatly refuses to accept that artificial
intelligence is possible at all, due to her insufficiently deep conception of
the nature of computers and simulation. Several others of my acquaintance accept
that computer simulation is useful for gaining insights into brain function, but
insist that, no matter how sophisticated the simulation, it can’t ever really
be conscious and can never be more than a pale imitation of the thing it
purports to represent. There are good ways and bad ways to approach computer
modelling of the mind, and unfortunately many people take the bad route,
provoking well deserved, but unnecessarily sweeping, criticism.
I should therefore like to differentiate between two kinds
or levels of simulation, ‘shallow’ and ‘deep’, because I think that many
people’s objections (and many fundamental mistakes in approach by researchers
into artificial intelligence) lie in an unwitting conflation of these two ideas.
But to begin with, I need to make some assertions about the nature of reality.
Intuitively, we tend to divide the universe up into
substance and form – tangible and intangible. We regard electrons and pot plants
as things – hardware – but paintings and computer programs as form – software.
Moreover, we tend to assign a higher status to hardware, believing that solid,
substantial things are real, while intangible ‘things’ are somehow not. Such a
pejorative attitude is deeply embedded in our language: tangible assets are
considered better than intangible ones; responsible people are ‘solid’, while
things that don’t really matter (!) are ‘immaterial’. But I suggest that this
distinction is completely mistaken: substance is really a kind of form; the
intangible is no less real than the tangible; hardware is actually a subset of
software.
Take an electron, for example. We intuitively think of
electrons as little dots, sitting on top of space. But an electron is really (in
my view anyway) a propagating disturbance in an electromagnetic field. It
persists because it has the property of self-maintenance. If you were to create
a set of randomly shaped ‘dents’ in the electromagnetic field, many of them
would lack this property and rapidly fade away or change form, but
electron-shaped dents would propagate themselves forward indefinitely, switching
between a magnetic phase and an electrical one in much the same way that
hemlines oscillate up and down as fashionable people try to differentiate
themselves from the crowd and the crowd simultaneously does its best to keep up
with fashion.
So an electron is not a dot lying on space, it is a
self-preserving dimple or vibration of space – it is therefore form, not
substance. And if electrons, protons and neutrons are form, then so is a table,
and so, in fact, are you. The entire so-called physical universe is composed of
different classes of form, each of which has some property that enables it to
persist. A living creature is a higher level of form than the atoms with which
it is painted. It even has a degree of independence from those atoms – a
creature is not a physical object in any trivial sense at all: it is a coherent
pattern in space through which atoms merely flow (as they are eaten, become part
of the creature and are eventually excreted).
I submit that everything is form, and that simple forms
become the pigment and canvas upon which higher persistent forms are able to
paint themselves. The universe is an inventive place, and once it had discovered
electrons and protons, it became possible for molecules to exist. Molecules
allowed the universe to discover life, and life eventually discovered
consciousness. Conscious minds are now a stable and persistent class of pattern
in the universe, of fundamentally the same nature as all other phenomena.
Perhaps there are patterns at even higher levels of organisation, of which we
are as oblivious as the individual neurons in our brains must be of our minds.
The relevance of this to computers is that they are also
capable of an endless hierarchy of form. Computers are supposed to be the
epitome of the distinction between hardware and software, but even here the
dichotomy blurs when you consider that the so-called hardware of a computer is
actually a pattern drawn in impurities on a silicon chip. Even if you regard the
physical silicon as true hardware, the computer itself is really software. But
leaving aside metaphysics and starting from what is conventionally regarded as
the first level of software – the machine instructions – computers are a
wonderful example of a hierarchy of form. At the most trivial level,
instructions clump together into subroutines, which give rise to modules, which
constitute applications, and so on. The particular class of hierarchy I have in
mind is subtly different from this one and has a crucial break in it, but the
rough parallel between the hierarchy of form in the ‘real’ universe and that
inside the software of a computer should be readily apparent.
Now consider this thought experiment: suppose we took an
extremely powerful computer and programmed it with a model of atomic theory.
Admittedly there are gaps in our knowledge of atomic behaviour, but our theories
are complete enough to predict and explain the existence of higher levels of
form, such as molecules and their interactions, so let’s assume that our
hypothetical model is sufficiently faithful for the purpose. The computer code
itself simply executes the rules that represent the generic properties of
electrons, protons and neutrons. To simulate the behaviour of specific
particles, we need to provide the simulation with some data, representing their
mass, charge and spin, their initial positions and speeds, and hence their
relationships to one another. If we were to provide data about the electron and
proton in a hydrogen atom, and repeat this data set to make several such atoms,
we would expect these simulated atoms to spontaneously rearrange themselves into
hydrogen molecules (H2), purely as a consequence of the mathematics
encoded in the computer program. If we were then to add the data describing some
oxygen molecules, and give some of them sufficient kinetic energy to start an
explosion, we would expect to end up with virtual water.
The computer knows nothing of water. It only knows how
electrons shift in their orbitals. But if the database of particles and their
relationships was large enough, and our initial model contained the rules for
gravity as well as electromagnetism, then the simulated water should flow. Water
droplets will form a cloud and rain will fall. If we add trillions of virtual
silicate molecules (rock) then the rain will also form rivers and the rivers
will cut valleys. Nothing in the code represents the concept of a valley – it is
an emergent consequence of the data we fed into our atomic simulation.
Given a powerful enough computer, and a hypothetical
scanning device that can extract the necessary configuration data from real
objects in our world, we would expect to be able to scan the atoms of a physical
clock, feed the numbers into the simulation, and watch the resultant virtual
clock tick. Now imagine what would happen if I turned such a scanner on myself.
Unless the Dualists are right, it seems to me that I would find myself copied
into the simulation. One of me would remain outside, marvelling at what had
happened, while the other copy would be startled to find itself inside a virtual
world of clocks and rivers, but otherwise perfectly convinced that it was the
same person who, a moment earlier, was standing in a computer room. The virtual
copy of me would strenuously assert that it was alive and conscious, and who are
we to disbelieve it?
Many people do disbelieve it. They insist that the copy of
me would just look as if it thought it was conscious. It wouldn’t be
real, they say, because it isn’t really made of atoms, it is only numbers. But
what evidence is there for this conclusion? I accept that the electrons and
protons are not real – they are merely mathematical models that behave as if
they were particles. But the molecules, the rivers, the clocks and the people
are emergent forms, which have arisen in exactly the same way that ‘real’
atoms, rivers and clocks arise. So what is the difference? An emergent
phenomenon is a direct consequence of the interaction of components having
certain properties and arranged in a certain relationship with one another. It
is the properties and the relationships that give rise to the phenomenon, not
the components. To my mind, molecules that arise out of the properties and
relationships of simulated particles are just as much molecules as those that
arise from so-called real particles.
Whether you accept this metaphysical argument about reality
doesn’t much matter. I partly wanted to draw a distinction between code-driven
programming and data-driven programming. In the above thought experiment, the
simulation became more and more sophisticated, not by adding more rules, but by
adding more data – more relationships. Indeed, the total quantity of data hasn’t
really increased. In the initial state, the program consisted of a few rules
describing atomic theory, and a vast database of particle positions and types,
all of which were initially set to zero. To add more sophistication to the
model, we simply made some of these values non-zero. We might equally have
started out with a database filled entirely with random numbers, in which case I
would expect the system to develop of its own accord, discovering new molecular
configurations that are stable, and losing those that are not. Eventually, such
a model might even discover life. The implications of data-driven programming
(which is related to object-oriented programming) are perhaps relevant only to
computer scientists, but it has a distinct bearing on computational
functionalism in AI, and lies at the heart of many people’s objections to the
idea that computers can be intelligent and/or conscious.
My assertion is that while computers themselves can’t be
intelligent or conscious, they can create an environment in which intelligence
and consciousness can exist. Trying to program a computer to behave
intelligently is practically a contradiction in terms – blindly following rules
is not normally regarded as intelligent behaviour. But if those blindly followed
rules are not actually the rules for intelligent behaviour, but rules for
atomic behaviour (or more practically, neural and biochemical behaviour),
then genuine intelligence, agency and autonomy can arise from them just as they
can arise from the equally deterministic and blind rules that govern ‘real’
atoms or neurons. To think otherwise is dualism.
Many AI researchers try to build computational models of
intelligence in the shallow, explicit way that I rejected above. Psychologists
develop computational models of intelligence too, and these models are generally
abstract, symbolic and relatively simple. Such abstract models may well be
helpful as theories of intelligence, but they don’t constitute
intelligence itself. If you were to embed an abstract psychological model of the
mind directly into a computer, you would merely have emulated the
behaviour of the mind as a shallow simulation, and it would almost certainly
fail to capture all of the mind’s features. A real mind emerges out of myriad
non-linear interactions, borne of the relationships between the properties of
its neurons and neuromodulator molecules. There may be severe limits on how much
this can be simplified. It could well be that the basic operating principles of
the brain are simple in concept yet not reducible in their implementation, and
no matter how convenient it might be to describe these principles in linguistic
or mathematical terms (as I will myself later), this doesn’t mean that the
abstraction is itself sufficient to implement a mind. An elephant is an animal
with a trunk like a snake, ears like fans and legs like tree-trunks. But
strapping a cobra and a pair of fans onto four logs doesn’t make an elephant.
To recap: a shallow simulation is a first-order
mathematical model of something. It is only an approximation and is merely a
sham – something that behaves as if it were the system being modelled.
But deep simulations are far more powerful and, I suggest, have a different
metaphysical status. Deep simulations are built out of shallow simulations. They
are second-order constructs, and their properties are emergent (even if they
were fully anticipated and deliberately invoked by their designer). Some
phenomena have to be emergent, and mere abstractions of them are no
better than a photograph.
To create artificial consciousness, I suspect we can’t just
build an abstraction of the brain. We have to build a real brain – something
whose implementation level of description is at least one step lower than that
of the behaviour we wish it to generate. Nevertheless, it can still legitimately
be a virtual brain, because (unless Penrose is right) we don’t need to
descend as far as the quantum level, only the neural one. Computers are
wonderful machines for creating virtual spaces in which such hierarchical
structures may be built. Trying to make computers themselves intelligent is
futile, but trying to use computers as a space in which to implement brains from
which intelligence emerges is not.
Everybody needs some body
Having suggested that synthesis might be more powerful than analysis at
extracting the core operating principles of the brain, and that the computer, as
a convenient simulation machine, might be capable of turning an implementation
of these principles into something from which a mind emerges, I need to discuss
how one might actually go about developing such a model. Where on earth do we
start?Perhaps the first point is that brains simply don’t
work in the absence of bodies. Absurdly futile attempts have been made to imbue
computers with such things as natural language understanding, without providing
any mechanism through which words might actually carry meaning. It is all very
well telling a computer that ‘warm’ means ‘moderately high temperature’, but
unless temperature actually has some consequence to the computer, such symbols
never find themselves grounded. Bodies and the world in which they are situated
provide the grounding for concept hierarchies.
Also, intelligence (if not also consciousness) has more to
do with learning to walk and learning to see than it does with playing chess and
using language, so having a body and its associated senses is important. People
often assume that getting a computer to see is a relatively simple problem, but
this is because they are only consciously aware of the products of their
visual system, not the raw materials it has to deal with. Point a video camera
at a scene and examine an oscilloscope trace of the camera’s output instead of
looking at the scene itself and suddenly the problem of interpretation seems a
lot harder. A creature with no sensory perception would be unlikely to develop
consciousness at all. Moreover, when we consciously imagine a visual scene we do
so by utilising our brain’s visual system. So vision and other such ‘primitive’
aspects of the brain are absolutely essential components of the conscious mind,
and therefore need to be understood and replicated.
Finally, to get at the fundamental operating principles of
the brain, we need to tackle as many aspects of brain function as we can at one
time, otherwise we will fail to spot the common level of description that unites
them. In any case, interaction between multiple subsystems is important for many
aspects of learning. For instance, our developing binocular vision may rely on
our ability to reach out and touch things to calibrate its estimates of
distance, while at the same time our capacity to reach out and touch things may
depend on our having binocular vision.
So
for all these reasons, the best approach to understanding the brain through
synthesis is probably to build a robot. Such a robot should be as comprehensive
as possible, to allow for multi-modal interaction and exploration of as many
aspects of perception, cognition and action as possible. In my case I decided to
build a humanoid (actually, anthropoid) robot, which I named Lucy (Figure 1).
Lucy has eyes, ears, proprioception and a number of other senses, plus a fair
number of degrees of freedom in her arms and head. My first attempt was built on
a very tight personal budget (whereas her nearest equivalent in academia cost
millions of dollars) and hence is rather limited. Recently, thanks to a NESTA
Dream Time award, I’ve been able to start work on Lucy MkII (Figure 2), which is
considerably more powerful and capable. It is tough for any machine to develop a
mind when it can’t see very well and can’t even reach to scratch its own nose,
so Lucy MkII’s extra capability will greatly improve my ability to test out
ideas over the coming years.
One important thing to mention about Lucy is the extent to
which I’ve tried to give her biologically valid sensors and actuators. Brains
evolved to receive signals from retinas and talk to muscles. They didn’t evolve
to listen to video signals and talk to electric motors. The signals leaving the
retina are not a bit like a video picture – they are distorted, blurred
(convolved), and represent contrast ratios rather than brightness, amongst other
things. These differences between biology and technology might be extremely
important. If we are to understand how the brain functions, it is crucial that
we try to speak to it in its own language. For that reason, much of Lucy’s
computer power is devoted to ‘biologifying’ her various sensors and actuators.
Castles in the air
Given a suitable robot and the appropriate computing methodology, we are just
left with the small problem of figuring out how the brain works. So how do we do
that, exactly?My own approach is to work from both ends of
the problem at once. Neuroscience and psychology have uncovered a dazzling array
of symptoms of brain activity: some detailed and some abstract, but all of them
bizarre. Somehow, all of these phenomena must contain clues about the underlying
mechanism, and the problem is essentially one of finding the level of
description that unifies them. For example, it is strange enough that
schizophrenics hear voices inside their heads, but who would have guessed that
many of them can also tickle themselves? The two features seem at first to be
quite unconnected. Yet if you regard both phenomena as disorders of
self/non-self determination, everything starts to make sense. The voices in a
schizophrenic’s head are presumably his own thoughts, but the vital mechanism
that normally masks such self-generated perceptions to prevent them from being
confused with external stimuli, has failed. Exactly the same logic explains
perfectly why most of us are unable to tickle ourselves while schizophrenics
can. It is by trying to find such unified levels of description that we can hope
to grasp the essence of what is going on underneath, and see glimpses of the
core mechanisms at work.
Simultaneously, one can begin from first principles and a
blank sheet of paper and work upwards from there. What does a brain have to do?
What are the key engineering problems it has to face? For instance, one problem
is the significant time it takes signals to travel from the senses through to
the deeper parts of the brain, especially when considerable processing is
involved. This observation alone is enough to suggest a raft of possible
mechanisms and consequent predictions, upon which evidence from neuroscience can
be brought to bear. In fact signal delays are the mainstay of my developing
theories of the brain, and why, I think, mammalian brains have evolved in the
way that they have.
To use this approach on such an incredibly hard problem
requires that one rely on skyhooks, to hold up otherwise unsupportable
hypotheses long enough to see where they might lead. Progress depends on
following up flaky ideas, stimulated by dubious and possibly absurd
interpretations of the evidence. For instance a whole chunk of my present work
grew out of a silly line of reasoning about why chemically staining one part of
the cortex makes it appear blobby while another area looks stripy. My logic is
almost certainly complete nonsense, but it led me on a fruitful path towards a
practical mechanism for visual invariance – an otherwise highly mysterious
phenomenon.
For this reason I find myself reluctant to record any of my
‘findings’ in a technical context yet, since so many of my ideas are still
suspended by skyhooks. This article is meant to be no more than a position paper
on behalf of the synthetic approach, not an exposition of a theory of the brain.
Nevertheless, it’s worth giving a general overview of the picture of Lucy’s
brain that is gradually emerging, since it demonstrates the synthetic method in
action (Figure 3), and also shows how counter-establishment an idea can become
when it is allowed to follow its own nose. The degree to which Lucy’s brain
mirrors natural brains remains to be seen, but all of my ideas are backed up by
evidence from neuroscience to a greater or lesser extent, and most have been
implemented and shown to work inside the real robot, at least up to a point.
There is no stereotypical model of the brain – no specific
theory to which all neuroscientists currently subscribe. Nevertheless, there is
a distinct underlying paradigm – a set of assumptions and models that are
held more or less unwittingly, more or less vociferously by many cognitive
scientists and lay people. For the sake of comparison with Lucy’s rather
dissident brain architecture, this paradigm can be shamelessly caricatured as
follows:
The paradigmatic brain is a factory, in which raw materials
flow in from the senses at one end, to be modified and assembled into intentions
in the middle, before squirting out the other end as motor signals. Very little
traffic of any significance passes in the other direction in this model. The
factory is also a fairly passive structure – more like a maze of empty corridors
through which nerve signals pass than a room full of noisy conversations that
continue even in the absence of input. It is very complicated, and has many
highly specialised departments, each with its own unique way of doing things.
These specialised structures are hard-wired by evolution to perform operations
on the data (as opposed to being consequences of the data themselves). The nerve
activity that runs through them is presumed to be finely detailed and precise,
more like the signals in a computer than ripples on a wobbly jelly. The system
is also taken to be largely reactive, whereby programmed actions are triggered
only by incoming sensory signals – any apparently pre-emptive action is
dismissed as illusory. In this paradigm, work on the ‘higher’ aspects of thought
must wait until all of the complex lower details have been fully understood, and
the ‘C-word’ is not to be uttered for at least another century.
Lucy’s brain, on the other hand, is a responsive balancing
act – a dynamic equilibrium between two opposing forces, which I’ve christened
Yin and Yang. These two signals flow in opposite directions along different
pathways in her brain, meeting at many points along the way, and playing an
important role in several distinct aspects of the brain’s activity and
development. Her brain begins as a simple repeated structure, onto which a
mixture of sensory experience and regular, internally generated, ‘test card’
signal patterns write a story of ever-increasing complexity and specialisation.
The specialisation in her cerebral cortex is not hard-wired but dynamically
created and maintained, much as frail ripples in the sand are defined and held
firmly in place by subtle regularities in the movement of waves. This map of
specialised areas is essentially a mirror of the world outside – a model, or
anti-model, of the world, whose deepest purpose is to undo all the changes that
the world wreaks upon Lucy, just as a photographic negative placed over its own
positive turns the whole image back to a uniform grey.
Like our own cerebral cortex, Lucy’s is divided into many
specialised regions, which in her case at least, have pulled themselves up by
their own bootstraps. Nerve activity dances on the surface of these regions in
huge sweeping patterns, and it is the shape of these patterns that carry the
information. Each dance takes place within a specific coordinate frame: retinal
or body coordinates towards the periphery, and more shifting, abstract spaces
deeper in. In Lucy, these coordinate frames are (or will be eventually) entirely
self-organised. The yin and yang streams carry with them information about other
coordinate systems through which they have passed, and the tension between these
flows generates a morphing effect, creating new intermediate frames between the
fixed peripheral ones. Some of this morphing occurs during development, but it
needs to be periodically maintained, and eventually this will happen while Lucy
sleeps – an alternation of slow-wave sleep with dream sleep is a crucial
component of her self-maintenance. I suspect that this basic mechanism for
stopping brains from falling apart is more fundamental, evolutionarily speaking,
than the more sophisticated mental processes that it makes possible, so perhaps
the Australian aborigines were right: perhaps consciousness arose out of the
Dreamtime and we dreamed long before we were awake.
Unlike the largely unidirectional, pipelined structure of
the stereotypical brain, Lucy’s neural circuitry is arranged like an inverted
tree, with both sensory and motor signals arising from the same leaves. Yin
flows in from the leaves towards the trunk, while yang flows outwards to the
leaves. Yin invokes yang, and yang invokes yin. The two spark off each other
like lightning dancing among clouds. Sensory signals flow inwards in search of
something to connect with – something that can make sense of them. Meanwhile,
expectation, attention and intention flow outward, looking for confirmation that
they are on the right track or are achieving their aims. Each affects the other.
The system is in a perpetual state of tension, between
sensory data on the one hand, which tell Lucy what is happening (or since nerve
signals travel slowly, what has just happened), and an opposing set of
hypotheses or intentions on the other, which provide a context for the
interpretation of these sensory signals and proclaim what Lucy expects to happen
next, or intends to happen next. The outgoing yang signals constitute a model,
forming a set of predictions on different timescales. Incoming yin confirms or
denies, pushes and pummels this interpretation of events, and meanwhile the
predictions fill in gaps or compensate for delays in the sensory data, creating
a running narrative of what is going on and allowing Lucy’s mental world to
remain one step ahead of the outside world.
Some of these yang signals can be described as attention,
some as expectation (sensory hypotheses) and some as intention. What you call
them depends only on their context: they are fundamentally the same thing. If a
yang signal erupts at a leaf that has control of eye movements, then one might
regard this as an attentional signal, swivelling the eyes to focus on a
potentially interesting or dangerous stimulus. But in other circumstances it
could be viewed as an expectation, turning the eyes to where something is
expected to appear next. In both cases we could reinterpret the signal
controlling the eyes as an intention to move them – essentially a prediction
about where they will soon be pointing.
The fundamental purpose of this interplay between
expectation and intention is to maintain Lucy in a contented and comfortable
state. If her needs change or the outside world changes, then these flows of yin
and yang seek to rebalance themselves, sometimes by changing Lucy’s internal
beliefs but usually by changing the world outside. At any moment there will be
two distinct but related states in Lucy’s brain, one represented by millions of
yin signals (her sensory state) and the other represented by equal numbers of
yang signals (her mental state). The system learns to react in such a way as to
keep these two states in balance. Lucy’s mental state, her internal narrative
about the world, is what I choose to call her imagination. In humans,
this is where consciousness resides.
A perfect match between her mental and sensory states is
nigh on impossible. Usually her mental state is trying to keep one step ahead of
her sensory state, making predictions or issuing intentions according to
context, and meanwhile her beliefs may or may not match reality. Different parts
of the two systems may be in greater or lesser correspondence. If incoming yin
signals are straightforward enough to be able to trigger corresponding yang
responses right out at the leaves, without requiring more advanced contextual
processing, then the trunk of the tree does not become involved. But since it
can never be silent, it freewheels. If this were to happen in a human being, I
would say that the person was daydreaming or thinking.
Sometimes daydreams lead to decisions, and decisions lead
to actions, and in this case yang signals from the trunk would stretch right out
to the leaves and cause change – something we might regard as a voluntary,
conscious decision, as opposed to a simpler unconscious response, were it to
happen in a human. The outgoing yang pathway is a limited resource. Because of
the very broad and diffuse way that Lucy’s nerve signals pass around her cortex
(crucial to the way they perform computations), it is only possible for one
‘thought’ at a time to traverse outwards along any given yang path. So when such
a yang cascade occupies one of the central trunk routes, Lucy’s whole body is
essentially given over to the same thought and no others at that level are
possible. Signals that reach right up to the trunk are thus ‘conscious’, because
they take over most of her mind, while those that cause more peripheral activity
can happen unconsciously, leaving the rest of the neural tree to float in a
daydream world of its own or deal with something else.
At the moment, not all of these ideas have been fully
implemented, and there is obviously a stupendous amount left to be understood
before I can see how to grow a whole brain in this way. Lucy I’s brain, before I
dismantled it to begin building Lucy II, had only a hundred thousand neurons
anyway – a millionth the size of a human brain. Moreover, despite having
developed some really strong intuitions about the basic operating principles
that should allow her brain to self-organise from scratch, I’m not yet able to
define these rules completely enough that all the messy details sort themselves
out, without me having to intervene. I accept that it is probably well beyond
the wit of one person to solve all of these problems, but for the moment things
are going well and I intend to keep up this absurd hubris, since I think that
non-disciplinary, holistically inclined individuals like me might actually have
more chance of success than large teams of specialists do. It is very hard for
ten people, with ten different mindsets, to examine ten completely different
aspects of a problem and yet still see the common ground.
But suppose that I really could solve all of these problems
eventually, perhaps twenty years from now. Suppose that I could implement the
rules for how my yin and yang circuits interact, in such a way that a complete,
neurologically plausible brain develops entirely of its own accord. Will Lucy
then be conscious? I simply don’t know. All I can say is that I might have
demonstrated an operational understanding of a machine that grows in such a way
that it comes to behave as if it were conscious. That may have to be
enough.
Meanwhile, work continues...
References
Chalmers, D. (1995). Facing up to the problem of consciousness. Journal
of Consciousness Studies, 2 (3), pp. 200-19.
Penrose, R. (1989). The Emperor's New Mind. Oxford University Press, NY
Turing, A.M. (1950) Computing machinery and intelligence. Mind,
LIX(236):433-460
|